Recent research by Yang Jiaqi, a doctoral student at Tongji University's Magic Lab, titled 'DFF: Decision-Focused Fine-tuning for Smarter Predict-then-Optimize with Limited Data,' has been accepted by AAAI 2025, a top international conference in the field of artificial intelligence. Yang Jiaqi was invited to attend the AAAI conference, where she gave an oral presentation and a poster presentation. This research is a phase result of the Didi Gaia collaborative project 'Resource Planning and Intelligent Decision-Making in Ride-Hailing Scenarios,' led by Researcher Su Zicheng, and received in-depth guidance from Dr. Liang Enming, Research Assistant Professor at City University of Hong Kong, Professor An Kun, and many algorithm experts from Didi Chuxing's ride-hailing MPT team.
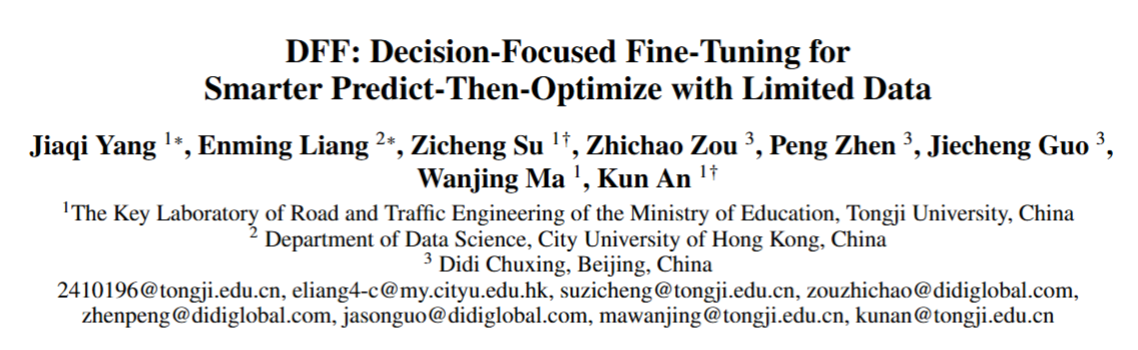
AAAI is an annual conference organized by the Association for the Advancement of Artificial Intelligence, one of the oldest and most comprehensive top international academic conferences in the field of artificial intelligence, and a Class A international academic conference recommended by the China Computer Federation (CCF). The 39th AAAI will be held in Philadelphia, USA, from February 25 to March 4, 2025. This year's conference received 12,957 valid submissions, of which 3,032 papers were accepted, with an acceptance rate of 23.4%. In addition, this research was successfully selected for the AAAI conference's Oral Presentation (a total of 600 papers were selected), with a selection rate of 4.6%.
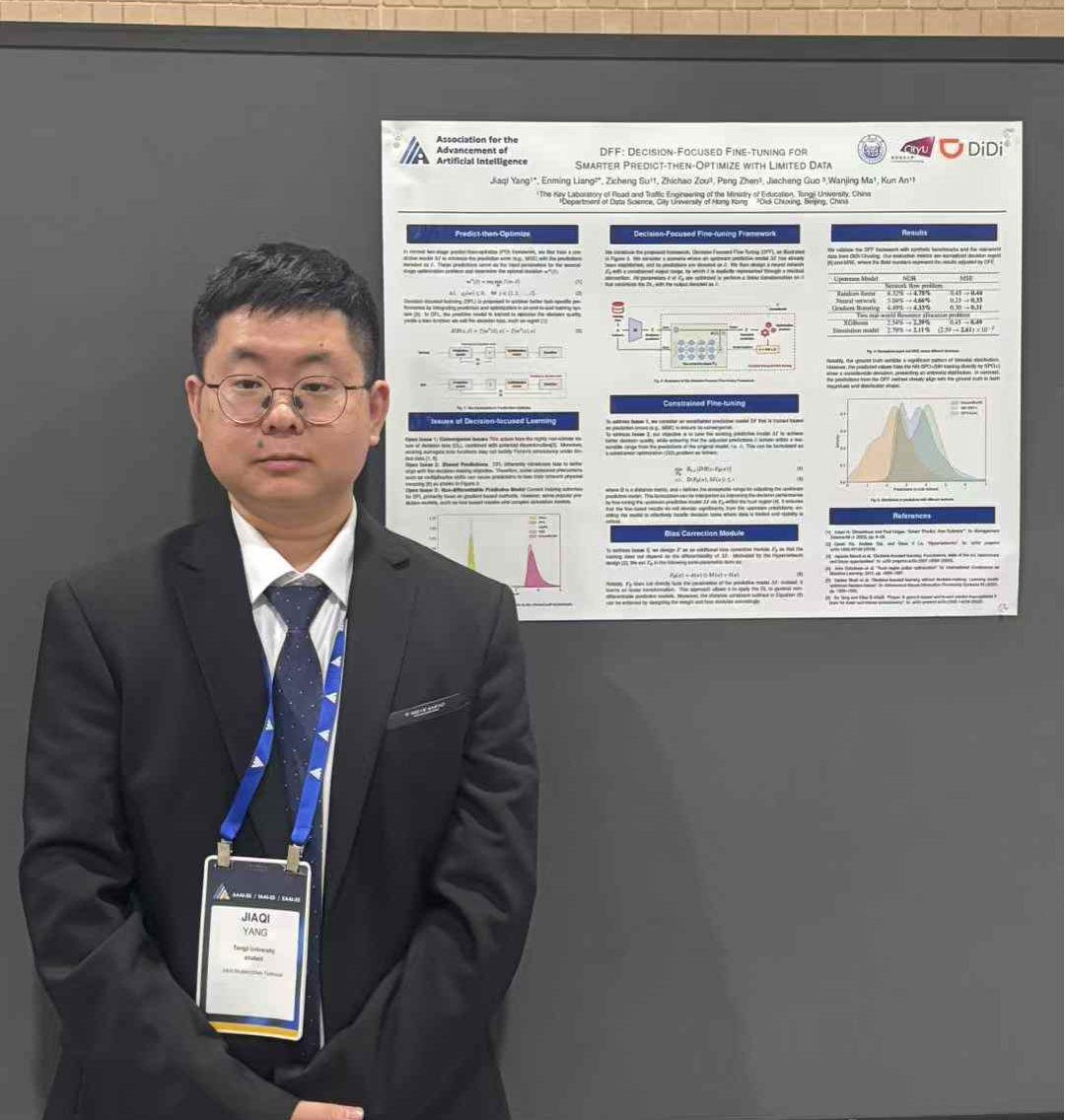
This research focuses on the improvement and innovation of Decision-Focused Learning (DFL) methods. By backpropagating the gradient of decision loss, DFL provides an end-to-end method for the traditional two-stage Predict-then-Optimize (PO) framework, improving the decision performance of PO systems. However, existing decision loss functions are difficult to converge with limited data, easily cause the prediction results to deviate from the original physical meaning, and cannot be applied to non-differentiable prediction models. To address these challenges, this research paper innovatively proposes a fine-tuning framework (Decision-focused Fine-tuning, DFF), which seamlessly embeds the DFL module into the existing PO paradigm by designing a bias correction module. At the same time, DFF is expressed as a constrained optimization problem, so that the output of the prediction model always stays within a predefined trust region. The method proposed in this paper has been extensively tested on generated data and real data from Didi Chuxing. The results show that DFF not only improves decision performance, but also strictly satisfies the fine-tuning constraints, and has good adaptability in multiple scenarios.
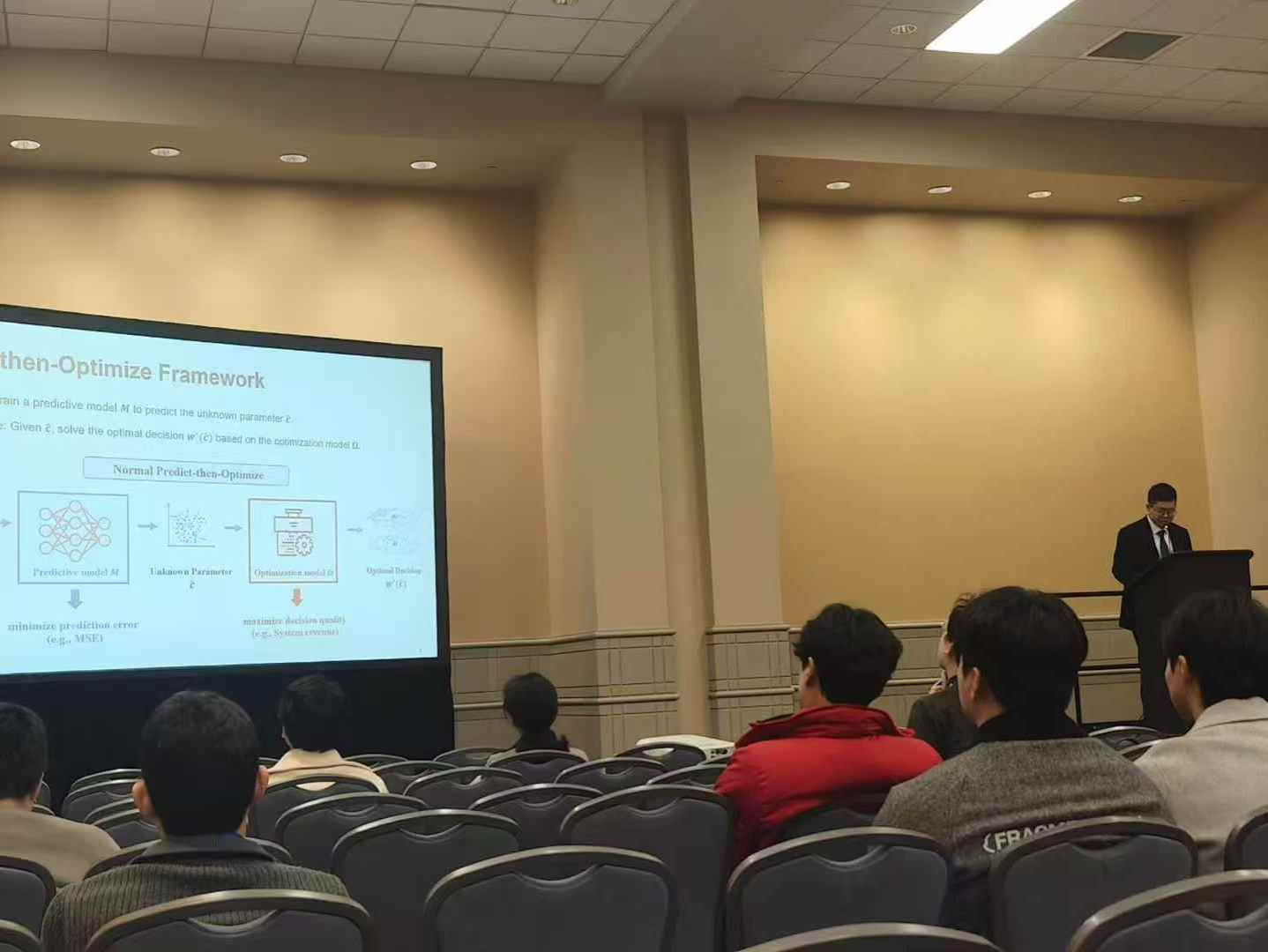